
J Cole Vintage T-Shirt, J Cole Shirt, Concert Tour Shirt, J Cole Tour 2022 T -Shirt sold by Gaurav Soni | SKU 40213139 | 55% OFF Printerval

Good Quality 2022ss Cole Buxton Fashion T Shirt Men 1:1 Cole Buxton Women Shirt Slogan Oversized Cotton T Shirts Men Clothing - T-shirts - AliExpress

Amazon.com: Rapper J Hip Hop Cole Shirts Men Cotton Short-Sleeve Round Neck T-Shirts Funny Graphic Tee Tops S : Clothing, Shoes & Jewelry
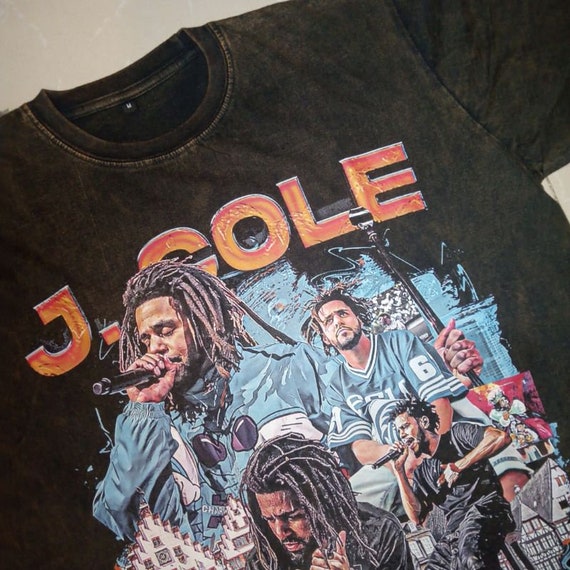